Winsorized Average: Calculation, Illustrations, and Significance
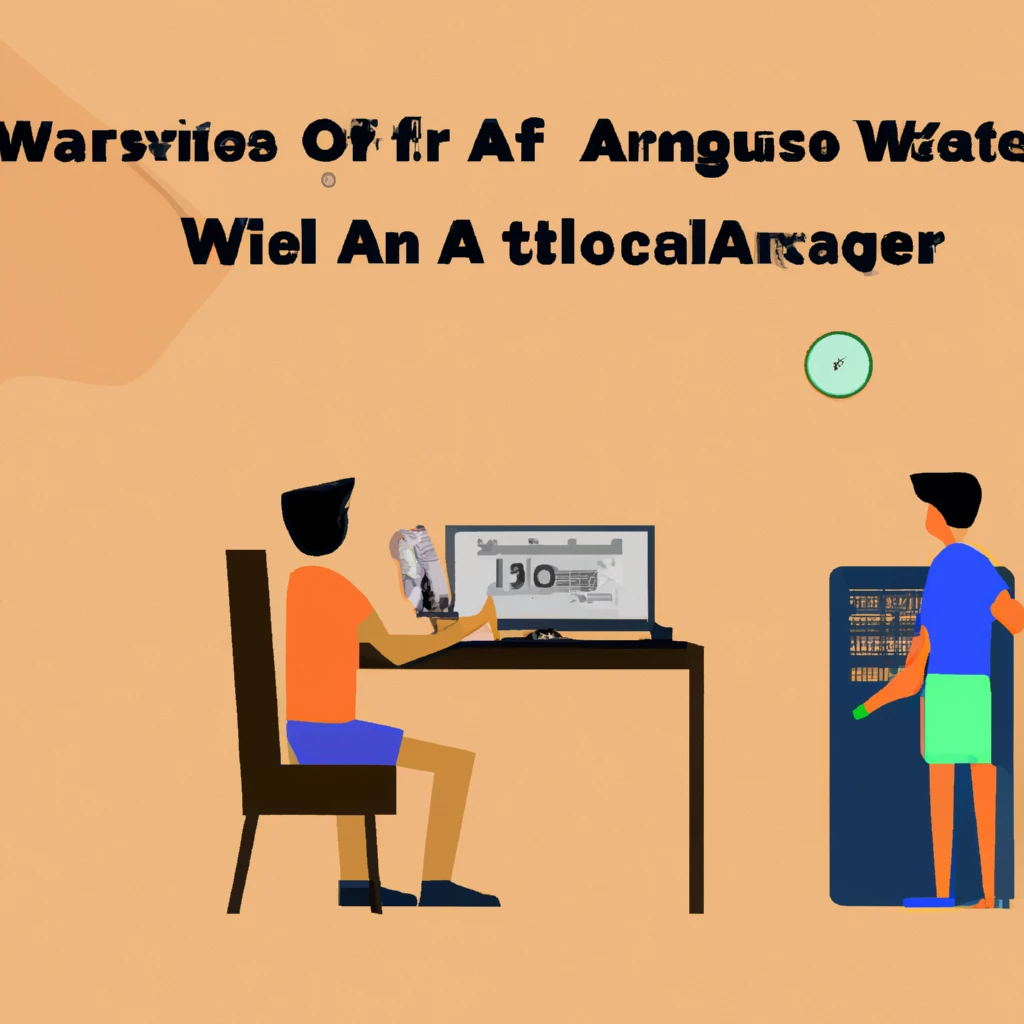
What Is the Winsorized Mean?
The Winsorized mean is an averaging technique that involves replacing the smallest and largest values in a dataset with values from nearby observations. This adjustment aims to reduce the impact of outliers or extreme values on the overall calculation. After replacing these values, the Winsorized mean is computed using the arithmetic mean formula.
### Key Points:
- The Winsorized mean replaces extreme values in a dataset to mitigate the effects of outliers.
- It is distinct from the trimmed mean, which involves removing data points instead of replacing them.
- Compared to the arithmetic mean, the Winsorized mean adjusts for outliers to provide a more robust estimate of central tendency.
Formula for the Winsorized Mean
Winsorized Mean = \frac{x_{n} \dots x_{n+1} + x_{n+2} \dots x_{n}}{N} where: n = The number of largest and smallest data points to be replaced by the observation closest to them N = Total number of data points
Winsorized Mean = x n … x n + 1 + x n + 2 … x n N where: n = The number of largest and smallest data points to be replaced by the observation closest to them N = Total number of data points
Two methods of expressing Winsorized means are the ‘kn’ winsorized mean where ‘k’ smallest and largest values are replaced, and the ‘X%’ winsorized mean where a specified percentage of values at both ends of the data are replaced.
### What Does the Winsorized Mean Tell You?
The Winsorized mean is less affected by outliers compared to the arithmetic mean, enhancing its robustness. However, in distributions with fat tails, the Winsorized mean’s impact may be limited due to the distribution’s high variability.
Winsorized means introduce bias but can improve analysis by reducing the influence of outliers, although some underlying data information is lost.
### Strengths of Winsorized Mean
Using Winsorized mean is advantageous in scenarios with outliers, skewed distributions, measurement errors, value fluctuations, and limited sample size, offering a more accurate measure of central tendency.
### Winsorized Mean Level
The Winsorization level determines the percentage of extreme values to replace, influencing the Winsorized mean’s effectiveness. Data domain knowledge plays a crucial role in setting the appropriate Winsorization level.
Understanding outliers and their impact aids in selecting the correct winsorization level. Higher levels are beneficial in minimizing outlier influence, while lower levels preserve original data characteristics.
### Winsorized Mean and Real World Situations
#### Financial/Investments
Market volatility in financial data can be better analyzed using Winsorized mean, reducing the impact of extreme price fluctuations and outliers.
#### Payroll/Salaries
Winsorized mean helps normalize skewed salary distributions by moderating the effect of abnormally high or low incomes, providing a more accurate representation of typical pay.
#### Health Care
Winsorization in medical data aids in analyzing health indicators without distortion from extreme readings, offering insights unaffected by outliers.
#### Education
Winsorized mean assists in evaluating educational data by mitigating the impact of abnormal test scores, ensuring a fairer assessment of performance.
#### Customer Satisfaction
Use of Winsorized mean in customer satisfaction ratings helps reduce the influence of extreme scores, allowing a more accurate depiction of overall satisfaction levels.
#### Environmental Data
Environmental data analysis benefits from Winsorized mean by providing a more representative average without distortion from extreme pollution levels.
### Example of How to Use Winsorized Mean
An example calculation of Winsorized mean showcases the process of replacing extreme values with their nearest observations to derive a more robust central tendency estimate.
Illustration of how Winsorized mean is applied with a numerical dataset demonstrates the impact of replacing extreme values on the final mean calculation.
Winsorized Mean vs. Other Measurements
Winsorized mean differs from traditional mean, trimmed mean, and median by better addressing outliers for a more accurate reflection of central tendency.
### Can Winsorized Mean Handle Multiple Outliers?
Winsorized mean can effectively manage multiple outliers by replacing a percentage of extreme values to maintain central tendency accuracy.
### Can Winsorized Mean Be Used with Non-Numeric Data?
Winsorized mean is not suitable for non-numeric data due to its nature of replacing numerical outliers for enhanced central tendency estimation.
### Does Winsorized Mean Preserve Data Variability?
Winsorized mean retains more data variability compared to trimmed mean, ensuring the preservation of the original data range and characteristics.
### How Does Winsorized Mean Impact Hypothesis Testing?
Winsorized mean aids in hypothesis testing by minimizing outliers’ influence, resulting in more reliable statistical outcomes, particularly in skewed or non-normal data scenarios.
The Bottom Line
Winsorized mean is a valuable statistical tool for replacing extreme values with more representative ones, offering a robust central tendency estimation while reducing the impact of outliers.