Types of Correlation Coefficients: Positive, Negative, and Zero
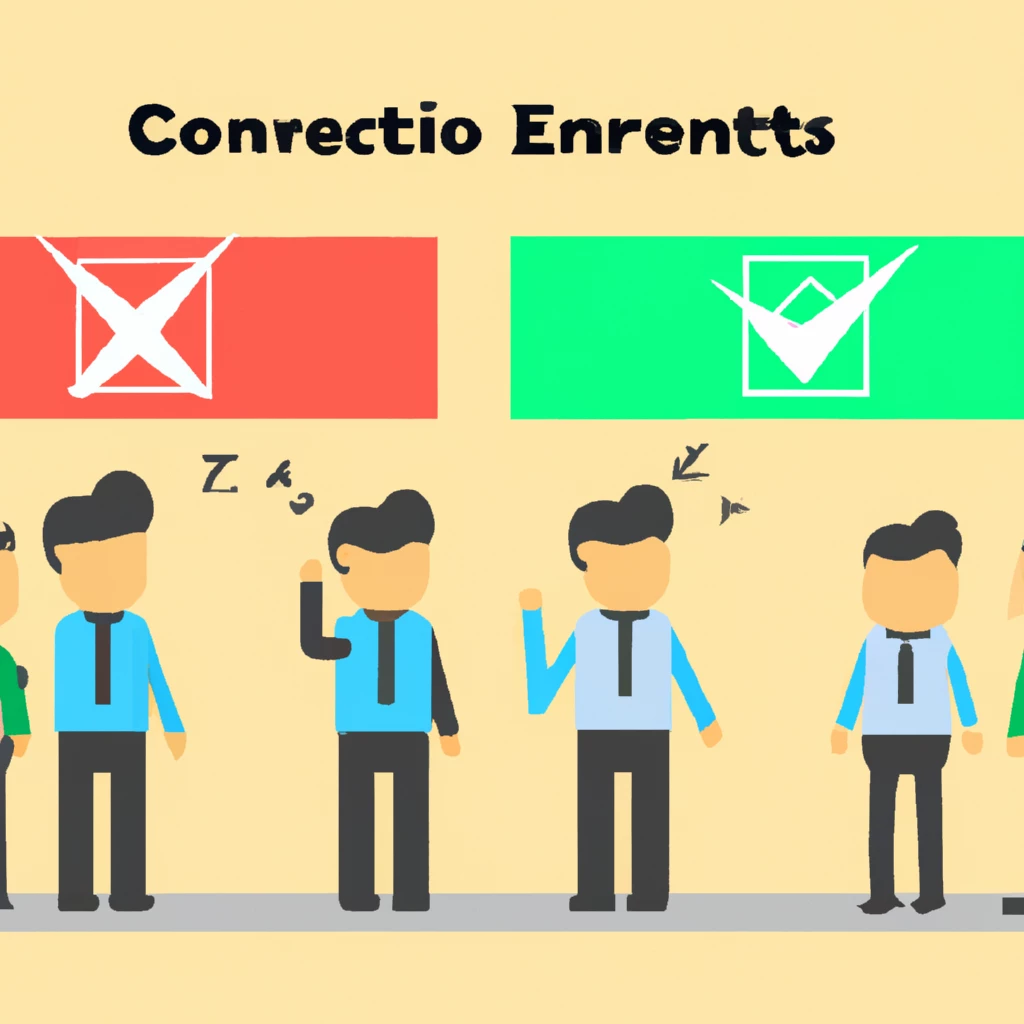
Correlation coefficients serve as crucial indicators of the strength of the linear relationship between two variables, denoted as x and y. When the linear correlation coefficient is greater than zero, it signifies a positive relationship between the variables. Conversely, a value less than zero indicates a negative relationship, while a value of zero implies no relationship between them.
This article delves into the importance of linear correlation coefficients for investors, elucidates how to compute covariance for stocks, and expounds on leveraging correlation for market prediction.
Understanding Correlation
The correlation coefficient (ρ) quantifies the extent to which two different variables’ movements are interrelated. Typically calculated using the Pearson product-moment correlation, this value gauges the linear relationship between the variables. However, in instances of non-linear relationships, this correlation coefficient may not be an ideal measure of dependency.
The correlation coefficient ranges from -1.0 to 1.0, defining a perfect negative correlation at -1.0 and a perfect positive correlation at 1.0. Values above zero indicate a positive relationship, while those below zero depict a negative one. A value near zero suggests a weak or negligible relationship between the compared variables.
When interpreting correlation, it’s crucial to note that correlation does not imply causation between the variables.
Correlation and the Financial Markets
In finance, the correlation coefficient aids in assessing the interrelation between two securities. When two stocks move together, the correlation coefficient is positive, whereas divergent movements result in a negative correlation.
A correlation coefficient of zero signifies a lack of linear relationship between the variables, but this is exclusive to linear relationships. Strong relationships can exist with a weak correlation coefficient if the relationship is non-linear. Values near zero (-0.1 to +0.1) imply minimal linear connection between variables.
For instance, a correlation coefficient of +.0008 between coffee and computer prices indicates an extremely weak correlation.
Investopedia / Hugo Lin
Calculating ρ
To derive the correlation coefficient, first calculate the covariance of the two variables in question. Subsequently, ascertain the standard deviation of each variable. Then, the correlation coefficient is computed by dividing the covariance by the product of the standard deviations of the two variables.
Standard deviation measures data dispersion from the mean, while covariance indicates how two variables change in unison. Utilizing software or statistical tools for this computation is advisable due to the time-consuming nature of manual calculations.
Correlation = ρ = (cov(X,Y))/(σXσY)
Positive Correlation
A positive correlation, denoted by a correlation coefficient above 0, signifies that both variables tend to move in tandem. At ρ = +1, the compared variables exhibit a perfect positive relationship, moving congruently in magnitude and direction.
A correlation coefficient closer to +1 intensifies the linear relationship’s strength. For instance, a correlation of +0.95 between oil prices and airfares indicates a robust positive correlation, where fluctuations in oil prices mirror airfare adjustments closely.
Furthermore, the correlation coefficient can be applied to comparing financial securities such as U.S. bank stocks and ETFs to gauge market trends and correlations.
Understanding the correlation between stocks and their industry aids investors in evaluating a stock’s performance relative to peers. The correlation coefficient is instrumental in comparing various securities, including bonds and ETFs, to assess mutual fund behavior concerning benchmark indices or other funds.
Negative Correlation
A negative correlation emerges when the correlation coefficient is below 0, indicating diverging movements between variables. A reading between 0 and -1 signifies opposite directions in which both securities move, with a correlation of -1 depicting a perfect negative correlation.
Despite the inverse relationship, the degree of negative correlation can fluctuate over time, rarely remaining consistent.
Examples of Negative Correlation
For instance, a strong negative correlation of -0.96 is observed between outdoor temperature and heating bills, illustrating that as temperatures drop, heating costs rise. In investing, negative correlations can aid in portfolio diversification to mitigate market volatility.
Negative correlations can play a pivotal role in portfolio construction, allowing investors to balance their holdings and reduce volatility risk by including negatively correlated assets.
Diversifying a portfolio by including assets with negative correlation can mitigate risk, as shown in a hypothetical scenario involving a balanced portfolio of stocks and bonds.
Comparatively, an all-equity portfolio would demonstrate higher volatility than a balanced portfolio due to differing returns during economic fluctuations.
Linear Correlation Coefficient
The linear correlation coefficient, calculated from data, evaluates the strength of the linear relationship between two variables, x and y. A coefficient near +1 or -1 suggests a profound relationship, while proximity to 0 denotes a weaker association.
Given the arduous nature of manual calculations, leveraging software or statistical programs for determining the correlation coefficient is recommended.
The Pearson Coefficient
The Pearson coefficient, similar to basic linear regression, gauges the linear association between variables. However, the Pearson coefficient focuses on quantifying the strength and direction of this association, without implying causation.
Ranging from +1 to -1, the Pearson coefficient indicates positive, negative, or negligible relationships between variables, devoid of causative implications.
Simple linear regression aids in forecasting by modeling the linear relationship between a response variable (y) and an explanatory variable (x).
Simplify linear regression computations through correlation calculations via software tools like Excel.
In financial analysis, correlation is crucial for portfolio standard deviation calculations, with software like Excel streamlining the process.
What Is the Linear Correlation Coefficient?
The linear correlation coefficient calculates the strength of the linear relationship between variables from given data.
What Is Meant by Linear Correlation?
The correlation coefficient, ranging from -1 to +1, indicates whether variables move in tandem or opposite directions.
What Is Considered a Strong Correlation Coefficient?
Generally, values closer to 1.0 or -1.0 imply robust relationships between variables, with values above 0.75 signifying high correlation and those between -0.3 and 0.3 indicating weak or negligible correlations.
The Bottom Line
Employed in determining relationships between investments and overall markets, the linear correlation coefficient plays a pivotal role in predicting stock market behaviors, particularly in the finance domain.
From mutual fund assessments to portfolio diversification, the correlation coefficient aids investors in making informed decisions and managing risks effectively.